Search Constraints
1 - 3 of 3
Number of results to display per page
Search Results
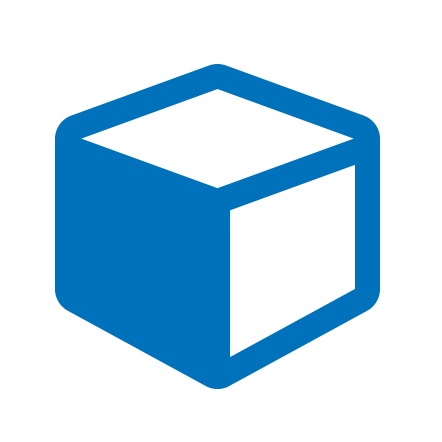
- Description:
- Isotopic data in this database includes 863 samples from 34 papers and three previously published compilations. For each sample, this database provides location, age, and reference information presented in the first columns. Locations are recorded in latitude and longitude (WGS84). The information about the location source uses the same criteria used for the elemental geochemical database (“GPS”, “Figure-Polygon” and “Figure-Point”). Age is provided according to the original source and includes two general scenarios: an age with uncertainty at 2σ level and a general estimation for the age with no associated error. Sm-Nd and Rb-Sr data are based on whole rock analysis. Lu-Hf data are based on zircon analysis. Sm-Nd data includes Sm and Nd in ppm, 147Nd/144Nd and 143Nd/144Nd in ratios, Nd uncertainties at 2σ level, and Nd values in the epsilon notation as presented in the data source. Rb-Sr data include Rb and Sr in ppm; 87Rb/86Sr, 87Sr/86Sr, and initial 87Sr/86Sr in ratios, and Sr uncertainties at 2σ level. Lu-Hf data includes 176Yb/177Hf, 176Lu/177Hf, and 176Hf/177Hf rations and their uncertainties at 2σ level, the initial 176/177Hf ratio, Hf values in the epsilon notation and Hf uncertainties at 1σ and 2σ level, all as presented in the data source. Uncertainties related to the data location and heterogenous data distribution should be considered. Samples for the two batholiths in Mongolia are concentrated in central Mongolia and include Sm-Nd and Lu-Hf data. In the Erguna and Xing’an magmatic provinces, available samples provide mainly Lu-Hf data which are relatively better distributed than in the other regions.
- Keyword:
- Mongolia, Mongol-Okhotsk Belt, magmatism, isotope geochemistry, and database
- Subject:
- Isotope geochemistry
- Creator:
- Ochir, Gerel, Lambart, Sarah, Lippert, Peter C., Henriquez, Susana, Johnson, Cari L., and Webb, Laura
- Owner:
- Kaylee Alexander
- Based Near Label Tesim:
- Mongolia, , Mongolia
- Language:
- English
- Date Uploaded:
- 10/27/2023
- Date Modified:
- 02/28/2024
- Date Created:
- 2020-05-01 to 2023-06-18
- License:
- Public Domain – This data is free of copyright restrictions (e.g. government sponsored data).
- Resource Type:
- Dataset
- Identifier:
- https://doi.org/10.7278/S50d-fraf-ds5g
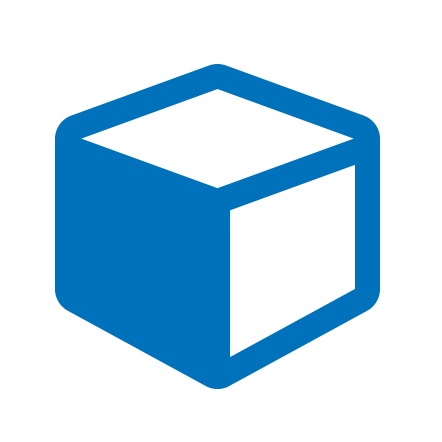
- Description:
- In the element database, major elements are reported in weight percent oxide (wt%). Trace element concentrations are reported in parts per million (ppm). Available lithologic information (“lithology” column) and the type of igneous sample (intrusive or extrusive in the “Sample-Type” column) were included. The name of the area or of the corresponding igneous body were included when available (“Location/Body-Name” column). The location of the samples is reported in decimal degrees (WGS84), however, uncertainties explained below must be considered. Coordinates were obtained from three different ways of presenting the information about the location. The three scenarios are distinguished as “GPS”, “Figure-Point”, and “Figure-Polygon” in the “Location-Type” column. Samples with a location in a coordinate system were transformed to decimal degrees (WGS84) and classified as “GPS”. Samples individually identified in a georeferenced geologic map were approximately located after georeferencing the map in Google Earth or ArcGis (“Figure-Point”). Samples identified with a polygon in a georeferenced map (through age, body name or unidentified sample locations), but without more detailed information were approximately located in the middle of the corresponding polygon after georeferencing the map in Google Earth or ArcGis (“Figure-Polygon”). Precise “GPS” locations were obtained for 358 analyses, and approximate locations were obtained for 428 analyses. The age information was organized using three categories: “Age-Approximation”, “Age-number”, and “Age-Error”. “Age-approximation” corresponds to the age information from original paper or from an additional reference detailed in the “Reference-Age” column. “Age-number” corresponds to the age reported in the original paper or previous compilation, or to the average age calculated from a given age range. “Age-Error” corresponds to the error presented in the original paper or previous compilation, or to half of the age range. Information about the methods, analyzed material and laboratory name was included when available. Lastly, the original data sources are available in the “Reference” column. References from previous compilations incorporated in this database are specified as “Compilation-Reference”. Additional references used for constraining the age are detailed in “Reference-Age” column. Data that were incorrectly reported (e.g., reporting average compositions instead of sample composition) or with anomalous trace element concentrations were filtered-out from the element database. Analyses from weathered or altered samples producing high total volatile content (LOI> 5 wt%) were removed. Samples with no available information to approximately locate them or to constrain their age were eliminated. Despite this screening process, the database suffers from uncertainties related to approximated ages and locations and variable information regarding the lithology, and availability of trace elements The inhomogeneity in this database is explicit and uncertainties related to the age and location should be carefully considered in any interpretation. The final compilation contains 787 geochemical analyses (major, minor and trace elements) and includes data from 36 studies.
- Keyword:
- Mongolia, Mongol-Okhotsk Belt, magmatism, whole-rock geochemistry, and database
- Subject:
- Geochemistry, Rare Earth elements, and Trace elements
- Creator:
- Ochir, Gerel, Lippert, Peter C., Henriquez, Susana, Webb, Laura, and Johnson, Cari L.
- Owner:
- Based Near Label Tesim:
- Mongolia, , Mongolia
- Language:
- English
- Date Uploaded:
- 06/26/2023
- Date Modified:
- 02/28/2024
- Date Created:
- 2020-05-01 to 2023-06-18
- License:
- Public Domain – This data is free of copyright restrictions (e.g. government sponsored data).
- Resource Type:
- Dataset
- Identifier:
- https://doi.org/10.7278/S50d-0phb-z0x0
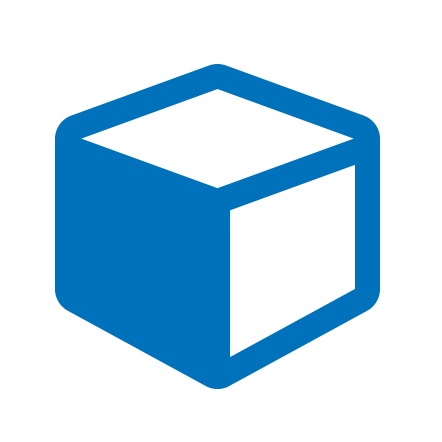
- Description:
- Classification of barrier island morphology stems from the seminal work of M. O. Hayes and others, which linked island shape to tidal range and wave height and defined coastal energy regimes (i.e., wave-dominated, mixed energy, tide-dominated). If true, this general relationship represents a process-based framework to link modern and ancient systems, and is key for determining paleomorphodynamic relationships. Here we present a new semi-global database of barrier islands and spits (n = 702). Shape parameters (aspect, circularity, and roundness) are used to quantify island boundary shape, and assess potential correlation with coastal energy regime using global wave and tide models. In adopting the original energy classification as originally put forth (i.e., wave dominated, wave-influenced mixed, tide-influenced mixed, tide dominated), results show that wave-dominated islands have statistically different mean shape values from those in the mixed energy fields, but the two mixed energy designations are not distinct from each other. Furthermore, each energy regime field contains a wide range of island shapes, with no clear trends present. Linear regression modeling shows that tidal range and wave height account for < 10% of the documented variance in island shape, a strong indication that other controls must be considered. Therefore, while energy regime distinctions can be used descriptively, their utility in predicting and constraining island shape is limited: barrier island shape is not indicative of coastal energy regime, and vice versa. Our analysis also demonstrates empirical scaling relationships among modern barrier islands for the first time, with implications for subsurface prediction. and This is the dataset of the Modern Barrier Island Database published in Mulhern et al., 2017 Marine Geology paper titled "Is Barrier Island Morphology a Function of Wave and Tide Regime?" with the DOI https://doi.org/10.1016/j.margeo.2017.02.016. If using this dataset please cite both the dataset and the paper.
- Keyword:
- paleomorphodynamic relationships, geology, barrier island, shallow marine, island shape, wave-dominated islands, shoreline morphology, Modern Barrier Island Database, and coastal geomorphology
- Subject:
- Geology
- Creator:
- Johnson, Cari L., Mulhern, Julia S., and Martin, John M.
- Owner:
- Julia Mulhern
- Language:
- English
- Date Uploaded:
- 09/08/2021
- Date Modified:
- 12/08/2023
- Date Created:
- 2015-01-01 to 2017-12-31
- License:
- CC BY NC - Allows others to use and share your data non-commercially and with attribution.
- Resource Type:
- Dataset
- Identifier:
- https://doi.org/10.7278/S50d-5pzj-r9vr